Advances in the Diagnosis of Urinary Tract Infection: A Narrative Review
Article information
Abstract
Urinary tract infections are among the most frequent bacterial infections, significantly impacting patient morbidity and healthcare resources. Prompt and accurate diagnosis is crucial to ensure effective treatment, prevent complications such as pyelonephritis or sepsis, and reduce inappropriate antibiotic use, contributing to antimicrobial resistance (AMR). Despite consensus across international guidelines from organizations, challenges persist, particularly in distinguishing true infections from asymptomatic bacteriuria or nonspecific symptoms, especially in older adults. Recent advancements in diagnostic technology have emerged to address these limitations, including molecular diagnostics, point-of-care testing (POCT), and artificial intelligence (AI)-driven predictive models. Molecular techniques, notably polymerase chain reaction, loop-mediated isothermal amplification, and metagenomic next-generation sequencing, offer enhanced sensitivity and specificity, rapid detection times, and comprehensive identification of pathogens and resistance profiles. POCT innovations, such as lateral flow immunoassays, enzymatic-based rapid tests, and novel biosensors, facilitate prompt bedside diagnosis, although specificity challenges remain. Meanwhile, AI and machine learning models demonstrate significant potential for risk stratification, prediction of infection, and improving antibiotics prescription practices yet face barriers related to validation, practical integration, and clinical acceptability. Despite promising developments, significant gaps remain, including limited real-world implementation evidence, high costs, and insufficient data from diverse populations. Further rigorous clinical studies, economic evaluations, and practical implementation assessments are urgently required. Addressing these research gaps could substantially improve patient outcomes, optimize antibiotic stewardship, and reduce the global burden of AMR.
HIGHLIGHTS
Urinary tract infections remain a major cause of antibiotic overuse and antimicrobial resistance due to diagnostic uncertainty, particularly in older adults and primary care. Recent advances—including molecular diagnostics such as polymerase chain reaction, loop-mediated isothermal amplification, and metagenomic sequencing—offer enhanced pathogen detection and resistance profiling, while emerging point-of-care technologies enable faster diagnosis in decentralized settings. Artificial intelligence models have shown potential in predicting infections and optimizing antibiotic use, although barriers to clinical adoption persist. Despite these innovations, widespread implementation is still challenged by high costs, infrastructure needs, and limited validation in real-world contexts, highlighting the need for tailored, cost-effective diagnostic strategies supported by robust clinical evidence.
INTRODUCTION
Urinary tract infections (UTIs) are among the most common bacterial infections, affecting individuals of all ages and genders, with a particularly high prevalence among women and older adults [1]. The diagnosis of UTIs is paramount, as a timely identification of the infection allows for appropriate treatment, minimizing complications such as pyelonephritis or sepsis and recurrent infections. However, the diagnosis must also be accurate as lower urinary tract symptoms may be nonspecific and lead to an inadequate prescription of antibiotics, leading to unwarranted adverse events and antimicrobial resistance (AMR) [2].
The diagnostic criteria for UTIs share several commonalities across the guidelines of renowned societies such as the Infectious Diseases Society of America, the European Association of Urology, and the Korean Association of Urogenital Tract Infection and Inflammation [3-5]. All three societies emphasize the importance of clinical symptoms such as dysuria, frequency, and urgency for diagnosing acute uncomplicated cystitis, while pyelonephritis is characterized by systemic symptoms such as fever and flank pain. For uncomplicated lower UTIs, urine culture as a gold standard for diagnosis is impractical, and in healthy women, the combination of two or more signs and symptoms can adequately identify a UTI in 90% of the cases [6], but these signs and symptoms have very low diagnostic yield in older populations [7]. Urinalysis, particularly for detecting pyuria and bacteriuria, is a valuable diagnostic tool, but a positive urine culture is required to confirm the diagnosis, especially in complicated or recurrent cases, as specificity may range from 42% to 90% [8]. Imaging is generally not recommended for uncomplicated UTIs but may be used in patients with persistent or severe symptoms [9]. Additionally, there is a consensus against screening and treating asymptomatic bacteriuria except in specific populations, such as pregnant women or those undergoing invasive urological procedures [10].
Even if these recommendations and diagnostic considerations have remained stable throughout the years, the threat of AMR is still high [11]. Estimates from the Global Burden of Disease indicate that UTIs are among the top four infectious syndromes related to loss in disability-adjusted life years [12]. The mechanisms linking AMR with inadequate diagnosis are numerous, but they include primarily the prescription of antibiotics to people with symptoms ultimately not attributable to infection (false positives), delayed prescription of antibiotics leading to the need to use high-potency antibiotics and for a more prolonged duration (false negative), and the inadequate selection of antibiotic due to poor identification or prognostication of target pathogens.
In recent years, novel diagnostic techniques have emerged to address these limitations, leveraging advances in molecular biology, biosensors, artificial intelligence (AI), and point-of-care testing (POCT). Molecular assays, such as polymerase chain reaction (PCR), allow for the rapid detection of bacterial DNA, offering higher sensitivity and specificity compared to traditional culture methods [13]. These techniques can identify fastidious and slow-growing organisms that might be missed in standard cultures. POCT devices have also gained traction, enabling rapid bedside diagnosis without the need for centralized laboratory facilities [14]. Integrating AI and machine learning (ML) into UTI diagnostics is another promising avenue, primarily in the form of predictive algorithms utilizing electronic health records (EHRs) and clinical parameters that can aid in risk stratification and treatment decision-making [15].
These advancements hold significant potential for optimizing UTI diagnosis accuracy and improving antimicrobial stewardship efforts. These advances will be clarified in the following sections, explaining the current status and supporting evidence.
This review aims to evaluate recent advances in the diagnosis of UTIs, specifically focusing on molecular diagnostics, POCT, and AI. Fig. 1 shows a comparative summary of the addressed diagnostic methods for UTI.
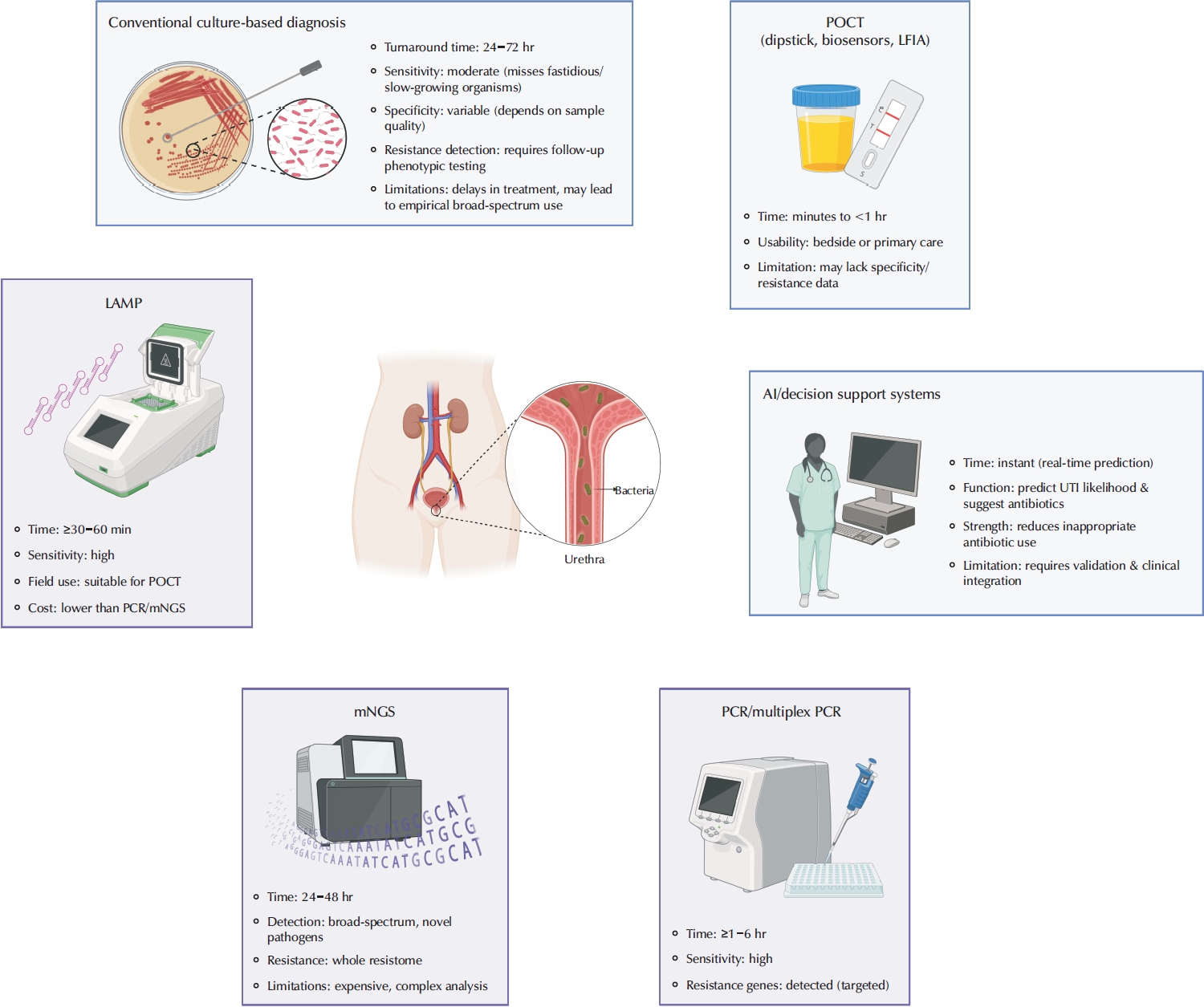
Comparison of conventional culture-based methods and emerging diagnostic technologies for urinary tract infection (UTI). POCT, point-of-care testing; LFIA, lateral flow immunoassay; LAMP, loop-mediated isothermal amplification; PCR, polymerase chain reaction; mNGS, metagenomic next-generation sequencing.
MOLECULAR DIAGNOSTICS
Molecular diagnostic techniques hold the promise to improve sensitivity, specificity, and turnaround time compared to traditional culture-based methods. These advances address limitations in conventional diagnostics, such as delayed results, false negatives, and the inability to detect certain fastidious or slow-growing pathogens [16].
One of the most significant advancements in molecular diagnostics for UTIs is the use of nucleic acid amplification tests, including the traditional PCR and loop-mediated isothermal amplification (LAMP) [17]. These techniques allow for the direct detection of bacterial DNA or RNA in urine samples, eliminating the need for bacterial growth.
1. Polymerase Chain Reaction
PCR, in particular, has demonstrated high, and sometimes superior sensitivity compared to urine culture, with the ability to detect low bacterial loads and identify polymicrobial infections that may be missed using traditional methods; however, this greater sensitivity may be accompanied by a lower specificity (59%) [17]. Moreover, multiplex PCR assays have been developed to simultaneously detect multiple uropathogens and resistance genes, providing a more comprehensive diagnostic approach, and their specificity might be higher than that of traditional PCR [18]. This is particularly useful for complicated UTIs, where identifying the correct pathogen and its resistance profile is critical for effective treatment.
2. Metagenomic Next-Generation Sequencing
Another key area of advancement is the development of metagenomic next-generation sequencing (mNGS), which provides a culture-independent approach to pathogen detection [19]. Unlike targeted PCR assays, mNGS can identify all microbial DNA or RNA present in a urine sample, allowing for the detection of rare, atypical, or novel pathogens, including Mycobacterium tuberculosis [20]. Furthermore, it can provide insights into host-pathogen interactions and AMR mechanisms. Although currently limited by high costs and data analysis complexities, mNGS has the potential to become a game-changer in UTI diagnostics, particularly in cases of recurrent or treatment-resistant infections.
In addition to bacterial identification, molecular diagnostics have advanced the detection of AMR genes [21]. Rapid molecular assays, such as PCR-based resistance gene panels, can identify key resistance determinants, including blaCTX-M/blaPER (extended-spectrum beta-lactamases), mecA (methicillin resistance), and carbapenemase genes [22,23]. Integrating these molecular tools into routine clinical workflows can help tailor antibiotic therapy more precisely, reducing unnecessary broad-spectrum antibiotic use and combating the growing threat of multidrug-resistant uropathogens.
3. Loop-Mediated Isothermal Amplification
LAMP technology has emerged as another promising molecular diagnostic tool due to its rapid amplification capabilities and minimal equipment requirements. Unlike PCR, which necessitates thermal cycling, LAMP operates at a constant temperature, making it more adaptable to POCT [24]. Studies have demonstrated its high specificity in detecting Escherichia coli. A recent study validated a simple, inexpensive, and portable distance-based paper device combined with a fluorescent closed-tube LAMP assay for rapid (≤3 hours) and reliable screening and semiquantification of E. coli infection in patients with UTI [25]. Another study developed and evaluated a point-of-care LAMP-based test panel for rapid detection of E. coli and antibiotic susceptibility in urine samples, demonstrating high sensitivity (95.4%) and specificity (96.1%), with the potential to guide immediate and appropriate antibiotic therapy for UTIs in the context of rising AMR [26-28].
4. Identifying AMR With Molecular Methods
PCR, LAMP, and NGS can identify genetic resistance in uropathogens, providing valuable resistome profiles to guide targeted antibiotic therapy for UTIs [29]. These techniques may be more sensitive than traditional culture-based methods and may be more practical by significantly reducing diagnostic time. However, they also have limitations. While they detect the presence of resistance genes, they cannot confirm gene expression or functional resistance, leading to potential false positives [30]. They may also miss resistance mechanisms driven by regulatory mutations, epigenetic changes, or enzymatic degradation not directly linked to known genetic markers. Moreover, molecular assays may fail to identify novel or emerging resistance genes outside their detection panels.
Despite these remarkable advancements, challenges remain in the widespread adoption of molecular diagnostics for UTIs. High costs, the need for specialized equipment, and the requirement for trained personnel limit accessibility, particularly in resource-constrained settings. Moreover, while molecular methods excel in pathogen detection, they do not provide direct information on bacterial viability or effective antimicrobial susceptibility, which are essential for clinical decision-making. Ongoing research aims to integrate molecular diagnostics with rapid phenotypic susceptibility testing, bridging this gap and further improving UTI management. Table 1 summarizes key studies evaluating molecular diagnostics, highlighting strengths and limitations.
POINT-OF-CARE TESTING
POCT promises to provide rapid, on-site detection of pathogens and biomarkers without the need for centralised laboratory facilities. Dipstick tests have been widely used as POCT due to their simplicity, affordability, and ease of use. However, they have low specificity, which may lead to overdiagnosis and overtreatment [31]. They can sometimes be used to rule out the diagnosis of UTI, although this depends on the pre-test probability [32]. Ultimately, they cannot differentiate between various pathogens and do not supply information regarding AMR patterns, usually requiring confirmatory testing in a laboratory.
1. Alternative to Traditional Dipsticks
Analogous to a home pregnancy test, lateral flow immunoassays have been created for UTIs. Instead of detecting human chorionic gonadotropin, these tests target bacterial antigens in urine. One example is RapidBac, a lateral flow kit that uses monoclonal antibodies to capture bacteria: it has one antibody against Enterobacteriaceae and another that broadly binds Gram-negatives and some Gram-positives. In essence, it is a ‘bacterial presence dipstick – if sufficient bacteria of the targeted groups are present, a test line appears with acceptable sensitivity and specificity [33].
Enzymatic-based rapid tests such as Uriscreen are designed to detect UTIs by identifying catalase activity in urine, an enzyme commonly produced by bacteria and leukocytes. Unlike dipstick tests, which rely on surrogate markers like nitrite or leukocyte esterase, Uriscreen detects the presence of active infection through a simple foam reaction, yielding results within one minute. Studies assessing its diagnostic performance have shown variable accuracy [14]. However, its specificity limitations stem from the fact that catalase is also present in non-infectious inflammatory conditions, potentially leading to false-positive results.
2. Dip-Slides and Culture Kits
Dip-slides are simple devices with agar media on a plastic paddle that can be dipped in urine and incubated outside a lab (even at room temperature or a small heating device). After overnight (16–24 hours) incubation at 35°C, they show colony growth, allowing semiquantitative estimation and valuable information: approximate colony count, an idea of which organism (via color/appearance), and even crude susceptibility (growth or no growth on antibiotic-containing sectors) [34]. These are some of the few POCTs that were evaluated in randomized controlled trials in primary care settings. These trials were largely negative, so their role in clinical care seems to be limited according to empirical evidence [35,36].
3. Biosensors and Emergent Technologies
Integrating biosensor technology in POCT has led to the development of highly sensitive and specific platforms for UTI detection. Electrochemical biosensors, which utilize microfluidic chips and nanomaterials, can detect bacterial metabolic byproducts, volatile organic compounds, and host response markers in urine [37].
The fidget spinner-based diagnostic device (Dx-FS) represents a novel instrument-free POCT, leveraging centrifugal forces to enable rapid bacterial detection and antimicrobial susceptibility testing. The device consists of a 3-dimensional-printed fidget spinner with microfluidic chambers, where spinning generates sufficient centrifugal force to concentrate bacteria from urine onto a nitrocellulose membrane for downstream colorimetric detection. The diagnostic process is completed within 50 minutes, significantly reducing turnaround time compared to conventional urine culture. Additionally, an integrated antimicrobial susceptibility testing function (Fidget-AST) exposes bacteria to antibiotics within the same system, allowing rapid phenotypic resistance assessment within 120 minutes. The Dx-FS system requires no electricity or external instrumentation [38].
A further innovation in POCT is the emergence of wearable and disposable UTI detection devices, such as smart incontinence pads and urinalysis patches [39]. These devices monitor urine composition and alert patients or healthcare providers when infection-related biomarkers are detected. Such technologies are particularly beneficial for individuals with recurrent UTIs, elderly patients, and those with neurogenic bladder disorders.
While POCT has made significant strides in improving UTI diagnosis, further research is needed to enhance the specificity of current devices, particularly in differentiating between colonization and infection. Additionally, efforts to integrate POCT with antimicrobial susceptibility testing are important for optimizing treatment strategies and combating antibiotic resistance. See Table 2 for a summary of the supporting evidence.
ARTIFICIAL INTELLIGENCE
AI and ML have transformed the landscape of healthcare diagnostics, offering powerful tools for the prediction, risk stratification, and personalized management of UTIs [15]. By leveraging large-scale datasets from EHRs, laboratory results, and clinical history, AI-driven models can enhance early detection, improve treatment decisions, and reduce unnecessary antibiotic use.
Prediction models powered by AI analyze patterns in patient data to estimate an individual's likelihood of developing a UTI. A recent systematic review by Shen et al. [40], published in 2024, identified 11 studies with 14 AI/ML models aimed at predicting UTIs. Only 2 of the studies were in outpatient settings, and mostly, they included inpatients or patients undergoing surgery. The meta-analysis of the performance of these models indicated a pooled area under the curve of 0.89 (95% confidence interval [CI], 0.86–0.92), sensitivity of 0.78 (95% CI, 0.71–0.84) and specificity of 0.89 (95% CI, 0.83– 0.93). Their analysis of the high heterogeneity (I2>99%) indicated that the reference standard definition (presence of culture) was a critical factor explaining the differing performance among models, as some employed diagnostic codes without culture confirmation. Furthermore, only five studies had external validations, so their findings may have limited applicability [40].
Interestingly, in less than a year, many prediction models using AI were published, both for the general risk of UTI and the risk of AMR and also in subpopulations, such as those with instrumentation of the urinary tract [41], nephrolithiasis [42] or surgery [43-45].
It is nonetheless sobering that although some of the novel predictive models are externally validated, explorations on their implementation are scarce. A recent large-scale study examined the real-world implementation of UTI Smart-Set (UTIS), an AI-driven decision-support tool designed to guide antibiotic prescriptions for outpatient UTIs [46]. UTIS incorporates ML predictions based on EHRs, individual patient history, and local resistance patterns, generating antibiotic recommendations aligned with clinical guidelines. In a cohort of more than 171,000 outpatient UTI diagnoses, the UTIS decision-support tool was used in 75,630 cases, with physicians accepting its recommendations in 66% of the instances [46]. Among the 19,287 cases with urine culture data available, adherence to UTIS recommendations significantly reduced antibiotic mismatch rates from 14.2% to 8.9%, particularly benefiting adult women over 50 years (with a mismatch reduction of 55.6%). Moreover, accepting UTIS recommendations led to an 80.5% reduction in ciprofloxacin prescriptions, a critical outcome given rising fluoroquinolone resistance [47,48]. These advancements in UTIS build upon prior ML algorithms that, in a retrospective study analyzing over 700,000 UTIs and more than 5 million antibiotic purchase records, showed that data obtained from a patient’s clinical history strongly predicts AMR patterns [49]. Importantly, tools such as UTIS lead to individualized antibiotic recommendations by integrating resistance forecasts with patient-specific features and local epidemiology, directly supporting physicians at the point of care. This novel approach not only enhances prescribing accuracy but also may contribute to the global effort to combat AMR by reducing inappropriate antibiotic use and preserving the efficacy of existing agents (Fig. 1).
Despite these encouraging outcomes, real-world implementation remains complex. The 66% adoption rate also means that 34% of clinicians declined to follow the recommendations of the tool [46]. Among other findings, the study shows that this reluctance stemmed from concerns about the reliability and accuracy of the algorithm, perceived inconsistencies with clinical experience, and the belief that the model did not fully capture individual patient nuances [46]. Moreover, some clinicians were hesitant to rely on algorithmic suggestions out of a desire to maintain their clinical autonomy [46]. These findings highlight that beyond technical performance, successful integration of AI tools requires transparent design, alignment with clinical reasoning, and trust-building strategies that support—rather than supplant—clinical judgment.
Recent articles assert that AI can potentially revolutionize predictive medicine [50]. A review on the topic highlighted the “efficacy of AI in diagnosing UTIs based solely on (urine) erythrocyte values in conjunction with symptoms such as suprapubic pain, pollakiuria, and urinalysis results” [51], which is the standard clinical diagnosis without the use of AI.
Finally, if AI requires large datasets and clinical information, including laboratory values and extensive testing, it may not reduce the diagnostic burden, especially in patients presenting with urinary symptoms in primary care. This is coupled with additional factors related to the trustworthiness of AI in clinical practice without extensive validation, transparency and buy-in from interest holders [52].
RESEARCH GAPS
Despite significant advances in diagnostic technologies for UTIs, several critical gaps remain. Future research should prioritize rigorous assessment of diagnostic accuracy through well-designed studies in diverse patient groups, including not only the postmenopausal women, elderly, pediatric, immunocompromised, and pregnant populations but also high-risk individuals such as those with recurrent infection and instrumentalization of the urinary tract [53]. Additionally, comprehensive economic evaluations are essential to determine the cost-effectiveness and real-world applicability of molecular diagnostics, point-of-care tests, and AI-based models [54,55]. Although methods such as mNGS, LAMP, and AI-based diagnostic tools offer high accuracy, their widespread adoption may be limited by cost, infrastructure requirements, and practical feasibility across diverse clinical settings. As shown by Bosmans et al. [54], sequential strategies involving basic tools—such as combining a patient history with a dipstick test—offered the best cost-effectiveness at lower willingness-to-pay thresholds, with strategies incorporating more advanced diagnostics (e.g., sediment or dipslide tests) adding accuracy at increasing cost. These findings underscore the need to design diagnostic pathways that balance clinical value with affordability and feasibility, particularly in primary care contexts. Moreover, the potential clinical benefit and feasibility of integrating these emerging diagnostic methods into routine practice must be further validated through robust implementation studies. Specifically, for AI-driven diagnostic tools, research should not only focus on the validation of predictive accuracy but also on their practical integration into clinical workflows, exploring their effectiveness in risk stratification, targeted treatment decisions, and, ultimately, their impact on patient outcomes and antimicrobial stewardship.
CONCLUSIONS
Advancements in diagnostic technologies for UTIs, including molecular diagnostics, POCT, and AI, have demonstrated substantial potential to enhance diagnostic accuracy and facilitate precise antimicrobial stewardship. Molecular tests and AI-driven predictive models have shown acceptable sensitivity, specificity, and speed compared to traditional methods. However, implementing these emerging diagnostics is hindered by practical challenges, including high costs, complexity, limited accessibility, and the necessity for specialized infrastructure and training. Moreover, despite promising evidence, current clinical guidelines do not yet endorse the routine use of these advanced diagnostic tools [8], underscoring the need for further validation, cost-effectiveness assessments, and practical integration strategies into clinical workflows. Future efforts should thus focus on robust clinical evaluation, standardization, and validation to enable evidence-based guideline updates and widespread clinical adoption, ultimately improving patient outcomes and combating AMR.
Notes
Funding/Support
This study received no specific grant from any funding agency in the public, commercial, or not-for-profit sectors.
Conflict of Interest
The authors have nothing to disclose.
Author Contribution
Conceptualization: JF, NM; Data curation: JF, NM; Formal analysis: JF, NM; Methodology: JF, NM; Project administration: JF; Visualization: NM; Writing - original draft: JF, NM; Writing - review & editing: JF, NM.